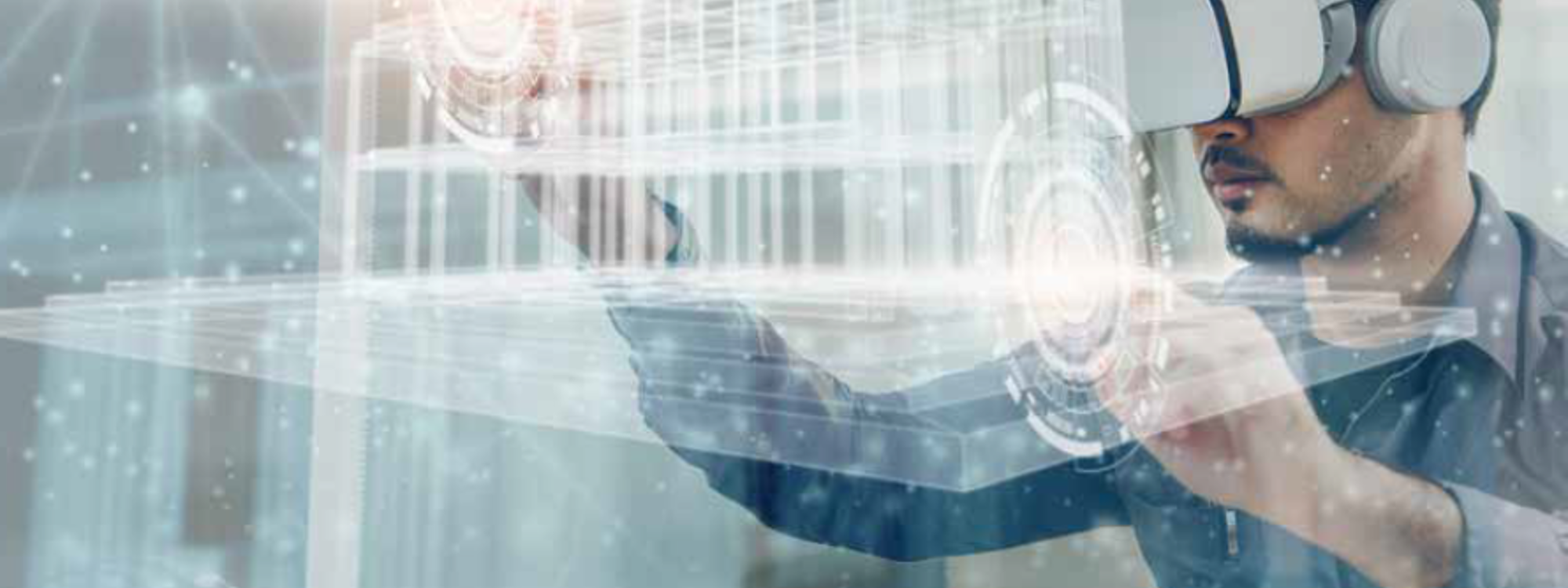
The use of Artificial Intelligence to enhance engineering design
Artificial Intelligence (AI) is the fastest growing emerging technology. Undertaken by machines, AI performs tasks undertaken by humans.
Challenge
AI is the simulation of human intelligence processes by machines, especially computer systems. It focuses on getting computers to do tasks that would normally require human intelligence.
Machine Learning (ML) is where machines learn by taking in data, analysing it, taking action and learning from the results of that action. Data used for AI and ML applications and projects is available in many different forms, from multiple sources, real-time and historic, which can be used to make engineering predictions and gain improved insight.
How can we use AI/ML in engineering to enhance design and simulation to reduce
the time, labour and cost of design and manufacturing processes?
How can we use this capability to our benefit to explore a wider range of design options for products and components, and discover patterns in data, speeding up decision making and freeing up engineers to focus on higher value tasks?
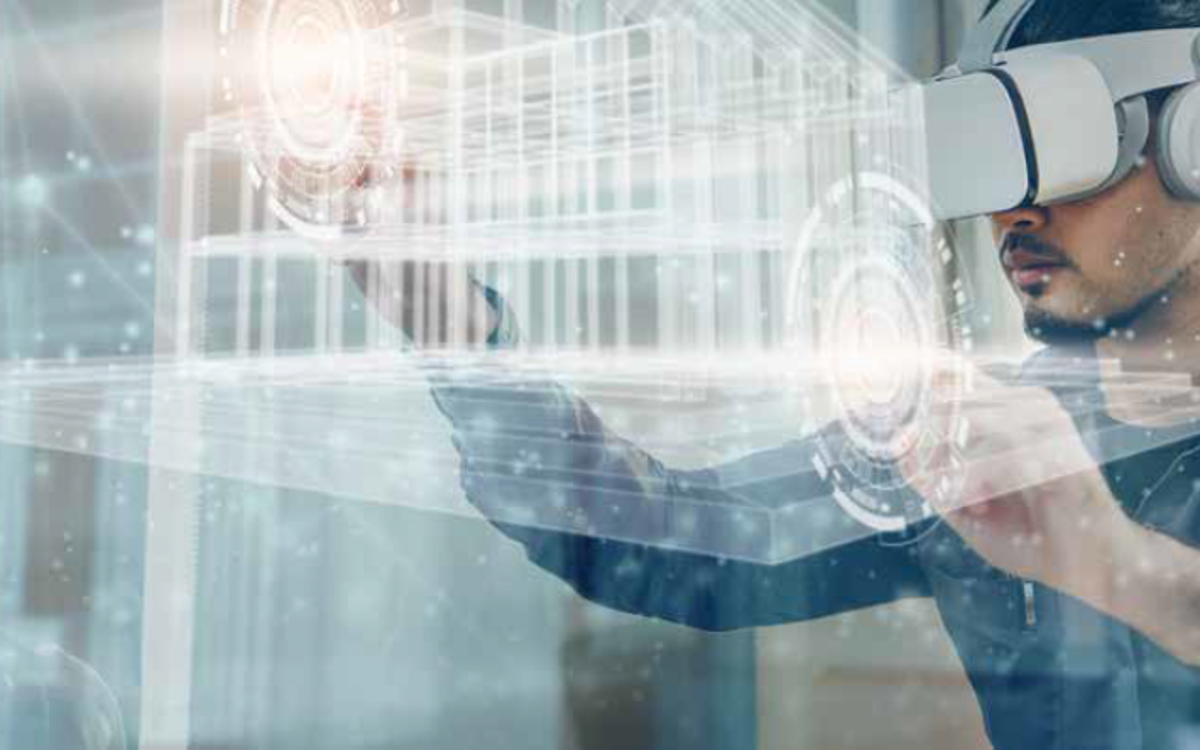
The specific aim of this industrial project was to investigate the challenges associated with the use of advanced digital methods, in this case AI/ML techniques to understand their value and limitations in the automation of aircraft design challenges. The project focused on determining the level of confidence that could be placed in decision-making and guidance from using an AI system trained using a training data set.
The key findings determined that the approaches used, given sufficient data, provided a high level of confidence in being able to make engineering predictions from one type of data set to another. The approaches utilised are capable of improving the accuracy of existing computer simulation methods used in the design of high value products.
With more reliable simulation, and improved ability to predict what a physical test may discover, this kind of approach may be able to reduce the amount of physical testing required by filling knowledge gaps or enriching a smaller physical dataset.
This technology therefore has the potential to create a significant cost saving in the development of new complex products through a reduction in the amount of physical testing required.
The results of this project provide further justification for the in-depth research and exploitation of AI/ML methods in digital engineering practice.
Detailed reports about the use of AI in the Digital Modelling Toolbox are available from CFMS.
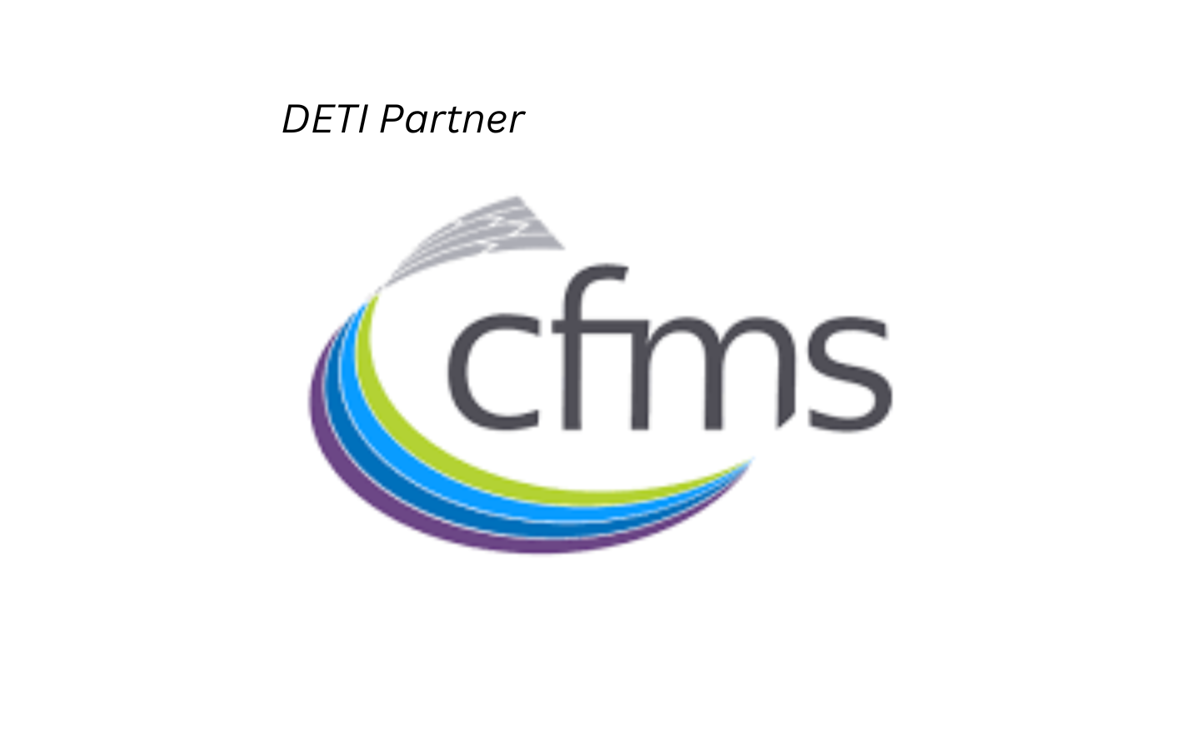