02 May 2024
Engineering experts at the National Composites Centre (NCC) have successfully developed a proof-of-concept machine learning tool that has the potential to drastically speed up the analysis of non-destructive testing (NDT). Completed as part of the NCC’s capability development programme, the project capitalises on one of the world’s largest composite data sets to explore data science solutions. The aim was to make NDT assessments quicker, less tedious, more reliable and more repeatable by reducing human factors.
Challenge
Ultrasonic testing (UT) uses high frequency sound waves to detect flaws inside a component. While one of the most common NDT options for composites, UT requires an interpretation of the signals received to infer information about the quality of the component being tested. This process of evaluation, analysis and reporting the location and severity of defects is often time-consuming, tedious, and requiring highly skilled verification experts in a shrinking pool of qualified inspectors. There is also the potential for variability from inspector to inspector within the set acceptance criteria. Larger, more complex, novel material parts with requirements for higher manufacturing rates are on the rise. Automated assistance for UT data evaluation could potentially help meet these ever-tightening customer timelines.
Innovation
Applying well-understood deep learning techniques to real inspection UT data, the team at NCC developed a tool that uses machine learning models to enable quicker and easier assessment of composite parts. The tool generates a handy pro forma report in approximately 5 minutes per square metre inspected, compared to an hour on average by human evaluation.
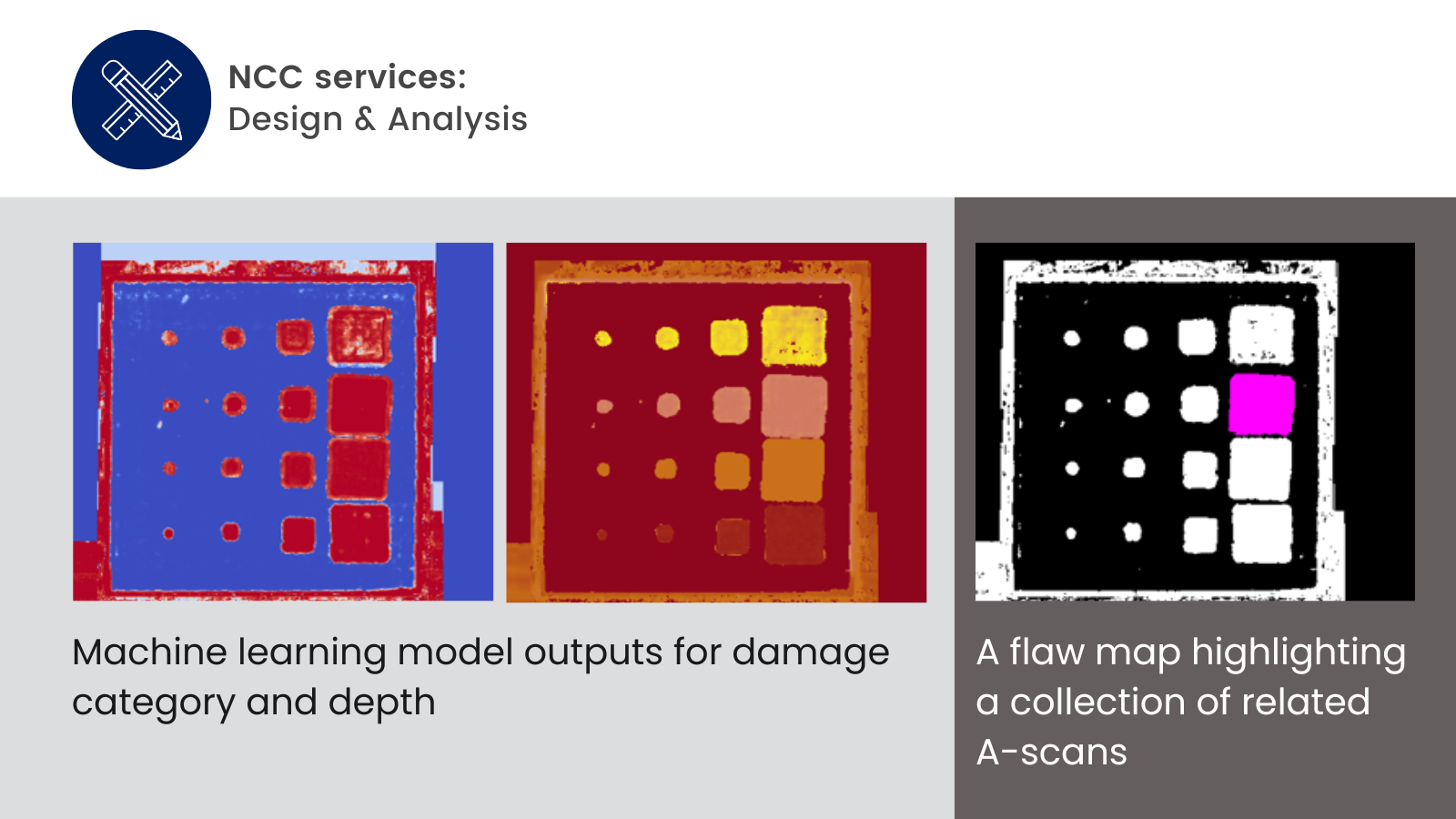
Results
The scope of work included manually tagging more than 84,000 A-scans from actual aerospace composite parts – building on what may be the largest data set of its kind in the world. Having designed a reusable tagging tool, verification experts were able to tag 450 composite samples per hour. This quality data is critical to data science, which helps develop and train high quality NDT models.
With machine learning, the automated tool was able to accurately detect and categorise defects in composite parts with an average accuracy of 90%. A second model was able to detect an average flaw location accuracy of 97% against unseen data.
The automated tool also converted the evaluation of ultrasonic inspection data into an actionable list. Delivered in a Microsoft Word format, the auto-generated report documented the maps of the full part. This includes damage category and depth for each location, as well as an enumerated list of flaws ordered by size.
Impact
The NCC’s proof-of-concept demonstrates the potential of machine learning in revolutionising how NDT data is assessed. By reducing the time required for data assessment, skilled operators can focus on the most value-added aspects of verification services and increase product throughput. In addition to promoting accuracy and repeatability, automated evaluation could also make it easier for engineers to identify defects, thereby contributing towards improved safety and reduced production costs in manufacturing.
Matt Scott, Chief Engineer for Technology at the National Composites Centre says:
"This project demonstrates how our collaborative approach to research and problem solving helps the UK industry anticipate and prepare for future challenges. Engineers combined their expertise in NDT and data science to deliver a game-changing solution capable of addressing an urgent gap in the NDT market. Expanding the potential of data science and machine learning in this manner also offers an opportunity for UK businesses to capitalise on national investments into artificial intelligence and high-performance computing."
Next steps
These preliminary findings were limited to idealised samples with simple geometry, in a specific material. Working towards industry trials, the NCC’s team of experts are currently expanding this NDT capability within the in-house composite integrity verification cell (CIVC). The cell enables faster and more repeatable NDT of more complex inspections. The scope of future work includes exploring fusing part geometry information and/or material characteristics. Other potential projects include co-simulation with machine learning to improve arbitrary part inspection.
Research partners interested in opportunities to collaborate on this work can contact [email protected] to express interest.